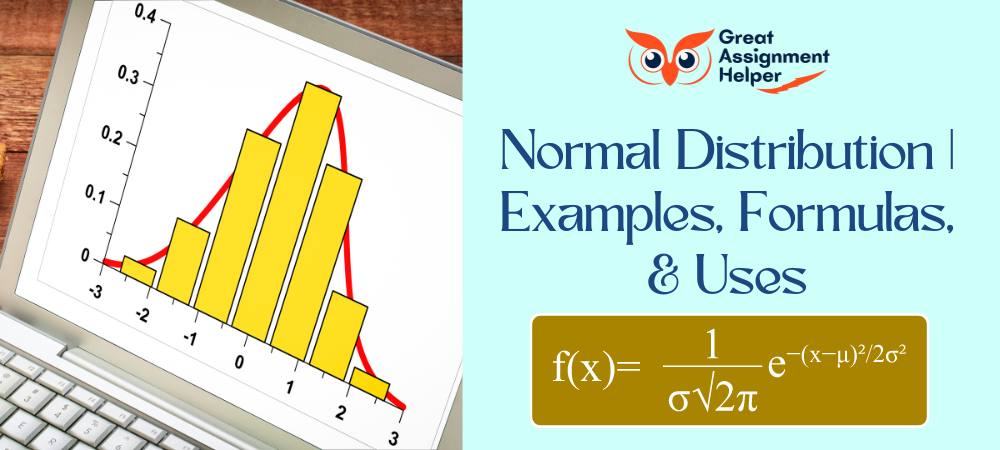
Listen To This Blog
Introduction
Diving into the world of statistics, the concept of normal distribution stands as a cornerstone, illuminating the path for analyzing data in various fields. This statistical phenomenon, often visualized as a bell-shaped curve, encapsulates a universe where data points cluster around a central mean value, revealing patterns and tendencies inherent to natural and social phenomena. From assessing student performance metrics to predicting market trends, the grasp of normal distribution equips professionals and researchers with the analytical tools needed to decode the complexities of their respective domains. By embracing this fundamental principle, one unlocks the ability to forecast outcomes, make informed decisions, and understand the variability inherent in data sets. This introduction sets the stage for a deeper exploration of how normal distribution functions as a pivotal element in the statistical analysis landscape, guiding insights and applications across a spectrum of disciplines.
What is normal distribution?
Normal distribution is a statistical concept that describes how data points are arranged in a pattern that resembles a bell-shaped curve. This pattern indicates that most data points are close to the mean (average) value, with fewer occurrences as you move away towards the extremes. Understanding normal distribution is crucial for analyzing data and making predictions. It plays a key role in determining how to calculate the probability of events, enabling researchers and analysts to assess the likelihood of various outcomes. By mastering this concept, one can effectively interpret data, forecast trends, and make data-driven decisions in diverse fields.
Properties of Normal Distributions
Normal distributions, pivotal in the statistical analysis landscape, exhibit several distinctive properties that make them uniquely valuable for data interpretation and probability calculation. These characteristics are:
- Symmetry: The distribution is perfectly symmetrical around the mean. This means the left and right halves of the curve are mirror images of each other.
- Mean, Median, and Mode Convergence: In a normal distribution, the mean (average), median (middle value), and mode (most frequent value) are all the same and located at the center of the distribution.
- Predictability: The distribution follows a predictable pattern of spread, allowing for the calculation of probabilities regarding the occurrence of certain values within the distribution.
- Standard Deviation: The spread of the distribution is determined by the standard deviation. A smaller standard deviation indicates that the data points are closer to the mean, resulting in a steeper curve, while a larger standard deviation shows that data points are spread out, leading to a flatter curve.
- Empirical Rule: Approximately 68% of data falls within one standard deviation of the mean, about 95% within two standard deviations, and about 99.7% within three standard deviations. This rule facilitates the estimation of probabilities and the identification of outliers.
- Asymptotic: The tails of the curve approach, but never touch, the horizontal axis. This indicates that there are always data points that exist far from the mean, although they are extremely rare.
Understanding these properties is essential for anyone looking to delve into data analysis, as they form the basis for interpreting data sets and calculating probabilities in a wide range of applications.
The Empirical Rule Explained
The Empirical Rule, a fundamental concept in statistics, serves as a quick reference for understanding the distribution of data points within a normal distribution. This rule posits that for a data set that follows a bell-shaped curve:
- Approximately 68% of all data points fall within one standard deviation of the mean. This segment captures the majority of occurrences, highlighting the central tendency of the distribution.
- Around 95% of the data is found within two standard deviations from the mean. This wider range encompasses almost all the data points, indicating a strong predictability in the spread of data.
- Finally, about 99.7% of data points lie within three standard deviations of the mean. This nearly complete coverage underscores the rarity of extreme deviations in a normally distributed data set.
The Empirical Rule is invaluable for analysts and researchers, providing a straightforward method to estimate the probability of occurrences within a given range, assess variability, and identify outliers in data. Its simplicity and effectiveness make it a cornerstone in statistical analysis and probability calculations.
Understanding the Central Limit Theorem
The Central Limit Theorem (CLT) stands as a cornerstone in the field of statistics, offering profound insight into the behavior of averages across different data sets. This theorem posits that regardless of the original distribution of a population, the sampling distribution of the mean will approximate a normal distribution as the sample size becomes sufficiently large. Key aspects include:
- Sample Size Significance: The theorem highlights that with an increase in sample size (typically 30 or more), the distribution of sample means tends toward a normal distribution, even if the population from which the sample is drawn is not normally distributed.
- Mean Convergence: It asserts that the mean of the sampling distribution of the mean is equal to the mean of the population.
- Standard Deviation Reduction: The standard deviation of the sampling distribution (also known as the standard error) decreases as the sample size increases, indicating more precise estimation of the population mean.
The Central Limit Theorem is pivotal for its implications in inferential statistics, enabling researchers to make inferences about population parameters based on sample statistics. It underpins many statistical procedures and tests, reinforcing the reliability of drawing conclusions from sample data, and is essential for understanding the distributional properties that allow for the estimation of probabilities and the formulation of confidence intervals.
What is the Standard Normal Distribution?
The standard normal distribution is a specialized case of the normal distribution, serving as a foundational concept in statistics for understanding and applying various analytical techniques. Here are the key characteristics that define it:
- Centered at Zero: Unlike a general normal distribution, which can have any mean, the standard normal distribution has a mean (μ) of 0. This central positioning simplifies analysis and calculation.
- Standard Deviation of One: The standard deviation (σ) is 1. This uniform scale means that the distribution provides a reference framework for comparing different normal distributions.
- Z-Scores: Values in a standard normal distribution are often referred to as Z-scores. A Z-score indicates how many standard deviations an element is from the mean. It standardizes different distributions for comparison.
- Probability Calculations: The standard normal distribution is crucial for calculating probabilities. Since it's well-defined, tables (Z-tables) and software can provide precise probability values for Z-scores.
- Universal Application: It allows for the transformation of any normal distribution to a standard form, enabling the use of standard tables and formulas for analysis, regardless of the original mean and standard deviation.
Understanding the standard normal distribution is essential for performing statistical tests, calculating probabilities, and making inferences about population parameters from sample data. It simplifies complex analyses by providing a common ground for comparison and computation.
Exploring the Normal Distribution Table
A normal distribution table, often referred to as a Z-table, is a valuable tool in statistics for finding the probability of a score occurring within a normal distribution and for assessing the probability that a score is below or above a certain value. Here's a simplified representation of how such a table might look, focusing on Z-scores (standard deviations from the mean) and their corresponding probabilities:
Z-Score (Standard Deviations) |
Probability (%) |
Cumulative Probability (%) |
---|---|---|
-3.0 |
0.13 |
0.13 |
-2.0 |
2.28 |
2.41 |
-1.0 |
15.87 |
16.15 |
0 |
50.00 |
50.00 |
1.0 |
84.13 |
84.85 |
2.0 |
97.72 |
97.89 |
3.0 |
99.87 |
100.00 |
Key Points to Remember:
- Z-Score: Represents the number of standard deviations a data point is from the mean. A Z-score of 0 indicates a score that is exactly at the mean.
- Probability (%): This column shows the likelihood of finding a score within the range up to the Z-score.
- Cumulative Probability (%): Indicates the probability of finding a score less than or equal to the Z-score, providing a running total from the left tail of the distribution up to the Z-score.
This table is instrumental in various statistical analyses, including hypothesis testing and confidence interval estimation, allowing for the quick assessment of probabilities associated with standard normal distributions.
Formula of the Normal Curve
The normal curve, emblematic of the normal distribution, is mathematically represented by the probability density function (PDF). This formula is pivotal for calculating the likelihood of a random variable falling within a specific range on the curve. The formula is expressed as:
2f(x)=σ2π1e−21(σx−μ)2
Where:
- f(x) is the probability density function for a given value of x,
- μ represents the mean of the distribution,
- σ is the standard deviation, indicating the distribution's spread,
- e is the base of the natural logarithm, approximately equal to 2.71828,
- π is Pi, approximately 3.14159,
- x is any value of the variable being measured.
This formula encapsulates the essence of the normal distribution, illustrating how data points are dispersed or concentrated around the mean (μ). The curve's shape—its peak at the mean and tapering tails—reflects the distribution's symmetry and the decreasing probability of values far from the mean. Understanding and applying this formula allows statisticians and researchers to delve into data analysis, predict probabilities, and model real-world phenomena with precision.
Normal Distribution Percentages
The normal distribution, a cornerstone of statistical analysis, is characterized by specific percentages that describe how data is dispersed around the mean. These percentages are crucial for understanding the distribution of data within different segments of the curve. Here's a breakdown of these key percentages, reflecting the distribution of data within standard deviations from the mean:
- Within ±1 standard deviation from the mean, approximately 68% of the data falls. This range captures the majority of outcomes and is the most densely populated segment of the distribution.
- Within ±2 standard deviations, about 95% of the data is covered. This wider range includes the vast majority of possible outcomes, leaving only 5% of occurrences in the tails.
- Within ±3 standard deviations, around 99.7% of the data is encompassed. This almost complete coverage signifies that values outside of this range are extremely rare and often considered outliers.
These percentages are fundamental to the empirical rule, offering a quick and intuitive way to estimate the probability of a random variable falling within a certain range. They enable researchers and analysts to assess the variability of data, identify outliers, and make informed predictions based on the distribution of data points around the mean.
Wrapping Up
In the intricate world of statistics, mastering concepts like normal distribution, the empirical rule, and the central limit theorem is crucial for academic and professional success. For students navigating these complex topics, seeking statistics assignment help can be a game-changer. Great Assignment Helper emerges as a reliable platform in this context, offering expert guidance and support. Whether it's understanding the nuances of probability calculations or interpreting data distributions, their team provides the assistance needed to excel. Turning to such resources not only clarifies challenging concepts but also enhances one's ability to apply statistical principles effectively in various scenarios.